Using Neural Networks to Deconvolute Complex Immune Landscapes 1
Using Neural Networks to Deconvolute Complex Immune Landscapes 1
Peter Westcott, Nathan Sacks, Thomas Westerling, Aiforia Technologies, Tyler Jacks
MIT Department of Biology, Koch Institute at MIT
This image shows high magnification light microscopy of a mouse colon tumor triple-stained for three important T cell subsets (CD8+ = black, CD4+ = green, CD4+/Foxp3+ = green with red nucleus), with these subsets annotated in the upper half of the image (CD8+ = orange circles, CD4+ = green circles, CD4+/Foxp3+ = purple circles) by a convoluted neural network that was rapidly trained using machine learning in collaboration with Aiforia Technologies.
Colon cancer cells have enlarged light blue nuclei arranged in glandular patterns, while interwoven areas of connective stromal cells have light blue nuclei that are thin and sickle-shaped, and are shaded in pink on the upper half of the image. CD8+ and CD4+ T cells are critical effectors of the adaptive arm of the immune system, while regulatory T cells (CD4+/Foxp3+) restrict the function of these effector cells. An increased ratio of CD8+ to regulatory T cells is an important clinical predictor of long-term survival and response to immunotherapy across many human cancers, but not colon cancer, for poorly understood reasons.
Explorations in faithful mouse models of colon cancer, such as the one featured here (induced “in situ” in the mouse colon from the relevant colonic epithelial cells of origin), can help provide critical insights into the functional importance of these different immune subsets. The broader goal of my research is to generate improved mouse models of colon cancer that better recapitulate the immune responses seen in human cancer, and that can be used as preclinical platforms to discover and test novel immunotherapies.
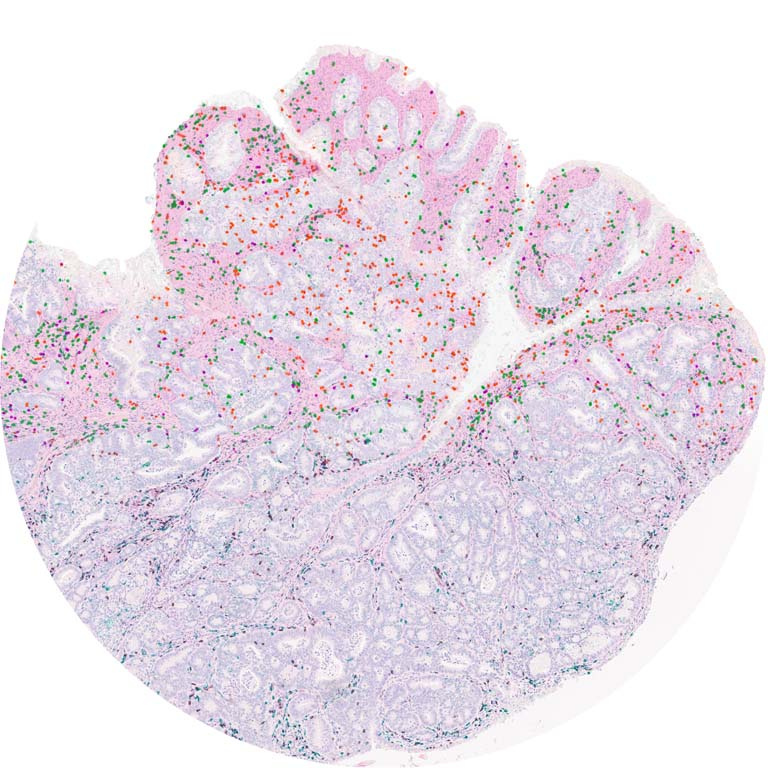